Mlops In The Age Of Generative Ai Charting A Course For Success
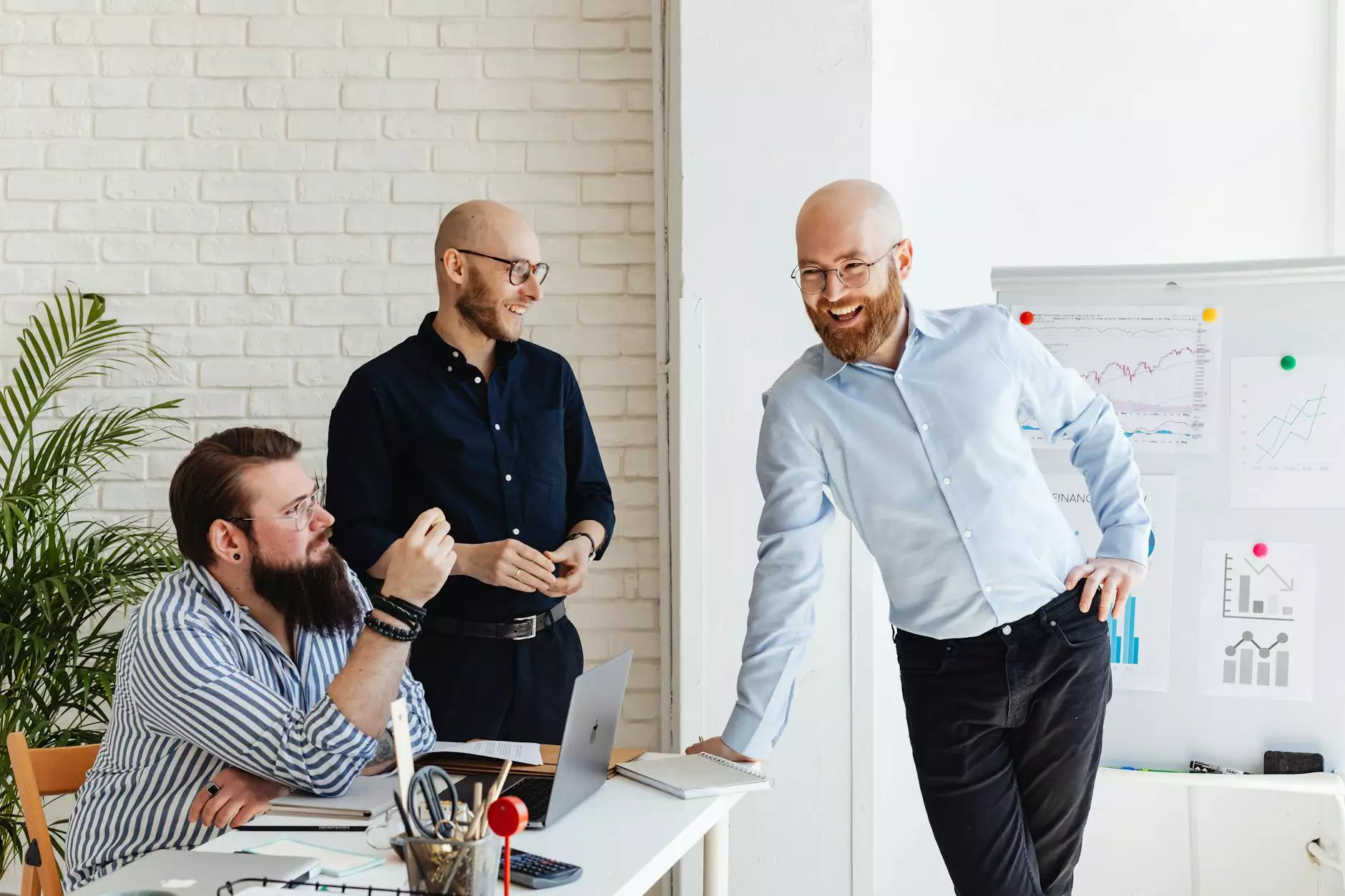
Introduction
Welcome to Workforce Outsourcing's insights blog, where we explore various topics related to business and consumer services. In this post, we will delve into the world of Mlops (Machine Learning Operations) in the age of generative AI and explain how businesses can chart a course for success by harnessing the potential of this innovative technology.
What is Mlops?
Mlops refers to the combination of machine learning and operations, focusing on the lifecycle management of AI models. It involves the processes, tools, and best practices necessary to enable seamless integration, deployment, monitoring, and optimization of machine learning models within an organization.
Generative AI and Its Impact
Generative AI, a subset of artificial intelligence, involves the creation of new content, such as images, videos, or text, by leveraging existing data patterns. It has revolutionized various industries, including healthcare, finance, and creative arts, by providing unique insights, generating personalized recommendations, and improving overall decision-making processes.
The Importance of Mlops in the Age of Generative AI
In the age of generative AI, Mlops plays a crucial role in helping businesses leverage the full potential of this technology. It enables organizations to effectively manage the development, deployment, and continuous improvement of generative AI models. By implementing Mlops practices, businesses can ensure the reliability, scalability, and interpretability of their AI solutions.
Charting a Course for Success with Mlops
To chart a successful course with Mlops in the age of generative AI, businesses should consider the following key steps:
1. Data Preparation and Annotation
The first step is to gather and prepare high-quality data that is representative of the problem or domain you are addressing. This involves data cleaning, transformation, and annotation processes, which are crucial for training accurate and reliable generative AI models.
2. Model Development and Training
Once the data is ready, businesses need to develop and train their generative AI models. This involves selecting the appropriate algorithms, tuning hyperparameters, and monitoring the model's performance to ensure its effectiveness in generating the desired outcomes.
3. Deployment and Integration
After successful model training, it's time to deploy and integrate the generative AI models into the production environment. This step requires careful consideration of infrastructure, scalability, and security aspects. Mlops practices ensure seamless integration and monitoring of the deployed models.
4. Continuous Monitoring and Optimization
A crucial aspect of Mlops is continuous monitoring and optimization of generative AI models. This includes monitoring the model's performance, detecting anomalies, and fine-tuning the model based on evolving business needs and changing data patterns. Regular updates and retraining are essential to maintain optimal model performance.
5. Ethical Considerations
It is essential for businesses to address ethical considerations when working with generative AI models. Ensuring fairness, transparency, and privacy in data usage and decision-making processes is vital. By adopting responsible AI practices, organizations can build trust with their customers and stakeholders.
Conclusion
As generative AI continues to shape industries and revolutionize business processes, leveraging Mlops becomes increasingly important to unlock the true potential of this technology. By following best practices for data preparation, model development, deployment, continuous monitoring, and ethical considerations, businesses can chart a course for success in the age of generative AI.